Using satellites to detect elephants from orbit
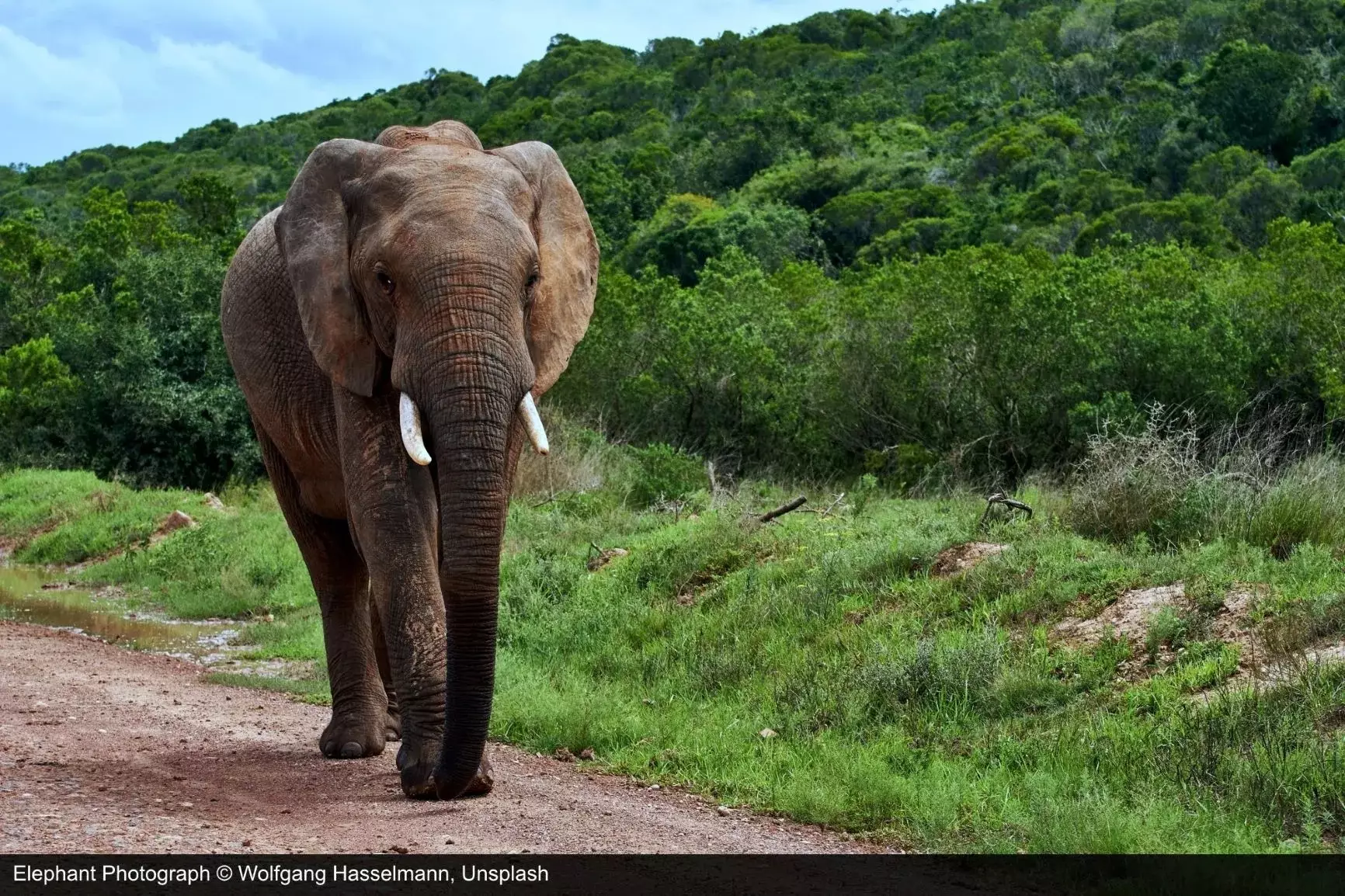
Researchers at the University of Oxford Wildlife Conservation Research Unit and Machine Learning Research Group, have combined satellite imagery with artificial intelligence capabilities, in order to detect and count African elephants (Loxodonta africana). The study has been published in the journal Remote Sensing in Ecology and Conservation in December 2020.
ELEPHANTS, SATELLITES AND AI
From stopping illegal hunting activities, to determining population densities or even help develop sustainable habitats for endangered species, satellites have proved on many occasions to be a viable detection method which directly helps wildlife conservation efforts worldwide.
When it comes to elephants, a species whose numbers are still decreasing in some areas in spite of the mammal being classified as endangered, reliable population counts are crucial for identifying and reducing the causes behind their decline. For this purpose, an Oxford team of researchers, in collaboration with Dr. Olga Isupova from University of Bath and Dr. Tiejun Wang from University of Twente, have developed a method to automatically spot and keep track of elephants from space. What is more, the study focuses on elephants found within the Addo Elephant National Park, a South African woodland savannah, which makes the detection of the animals all the more difficult due to the high heterogeneity of the environment.
To achieve its goal, the method integrated the highest resolution commercially accessible satellite data, obtained from the WorldView-3 and 4 spacecrafts (Maxar Technologies), with a Convolution Neural Network (CNN) deep learning model. In detail, the team has used 11 images captured by the two spacecrafts during different seasons between 2014-2019. Furthermore, they have identified 1125 elephants on the images, which were subsequently labeled and used as the training dataset for the deep learning algorithm.
The detection accuracy of the model (F2 score) was compared to that of human identification capabilities and it was concluded that satellite remote sensing and AI can detect and count these mammals just as well as humans. As an illustration, in heterogeneous landscapes the CNN model obtained an accuracy detection of 0.78, while the human labels averaged an F2 score of 0.77. On the other hand, in homogeneous areas the F2 scores the deep learning algorithm and human observation accuracy obtained are 0.73 and 0.80, respectively.
Additionally, the same model with no additional training data was tested on a satellite image acquired by a lower resolution spacecraft, overlooking a different study site, the Maasai Mara National Reserve in Kenya. Under these circumstances, the model still detected over half of the elephants and obtained an F2 score of 0.57, which demonstrates the generalisability of the method.
Given these points, the study illustrates the feasibility of using satellites and AI as a wildlife surveying tool, facilitating conservation endeavours worldwide.
References
1. Duporge, I. et al. 2020. Using very‐highresolution satellite imagery and deep learning to detect and count African elephants in heterogeneous landscapes. Remote Sensing in Ecology and Conservation, [online] Available at: https://zslpublications.onlinelibrary.wiley.com/doi/full/10.1002/rse2.195 [Accessed 20 January 2021].
2. Phys.org. 2021. Spotting Elephants From Space: A Satellite Revolution. [online] Available at: https://phys.org/news/2020-12-elephants-space-satellite-revolution.html [Accessed 20 January 2021].
3. Skyrora.com. 2020. Fighting Against Poaching From Space | Skyrora. [online] Available at: https://ad.skyrora.com/post/fighting-against-poaching-from-space [Accessed 20 January 2021].
4. Skyrora.com. 2020. Space Technology And Ridgway's Rail Habitat Protection | Skyrora. [online] Available at: https://ad.skyrora.com/post/space-technology-and-ridgways-rail-habitat-protection[Accessed 20 January 2021].
5. Skyrora.com. 2020. Utilising Satellite Technology For Wildlife Conservation | Skyrora. [online] Available at: https://ad.skyrora.com/post/utilising-satellite-technology-for-wildlife-conservation[Accessed 20 January 2021].
6. Skyrora.com. 2020. Whales From Space |Skyrora. [online] Available at: https://ad.skyrora.com/post/whales-from-space [Accessed 20 January 2021].